Barbara Di Eugenio
Engaging the Patient in Healthcare: Summarization and Interaction
Effective and compassionate communication with patients is becoming central to healthcare. I will discuss the results of and lessons learned from three ongoing projects in this space. The first, MyPHA, aims to provide patients with a clear and understandable summary of their hospital stay, which is informed by doctors’ and nurses’ perspectives, and by the strengths and concerns of the patients themselves. The second, SMART-SMS, models health coaching interactions via text exchanges that encourage patients to adopt specific and realistic physical activity goals. The third, HFChat, envisions an always-on-call conversational assistant for heart failure patients, that they can ask for information about lifestyle issues such as food and exercise.
All our work is characterized by: large interdisciplinary groups of investigators who bring different perspectives to the research; grounding computational models in ecologically valid data, which is small by its own nature; the need for culturally valid interventions, since our UI Health system predominantly serves underprivileged, minority populations; and the challenges that arise when dealing with the healthcare enterprise.
Short Bio: Barbara Di Eugenio is a Professor and Director of Graduate Studies in the Computer Science department at the University of Illinois Chicago. There she leads the NLP laboratory (http://nlp.cs.uic.edu/). She obtained her PhD in Computer Science from the University of Pennsylvania (1993). Her research has always focused on the pragmatics and computational modeling of discourse and dialogue, grounded in authentic data collection on the one hand, and in user studies on the other. The applications of her work run the gamut from educational technology to human-robot interaction, from data visualization to health care. Dr. Di Eugenio is an NSF CAREER awardee (2002); a UIC University Scholar (2018-2020); and a Zenith Award recipient from AWIS, the Association for Women in Science (2022). She has been the editor-in-chief for the Journal of Discourse and Dialogue since 2019. She is very proud to have graduated 15 PhD and 32 Master’s students.
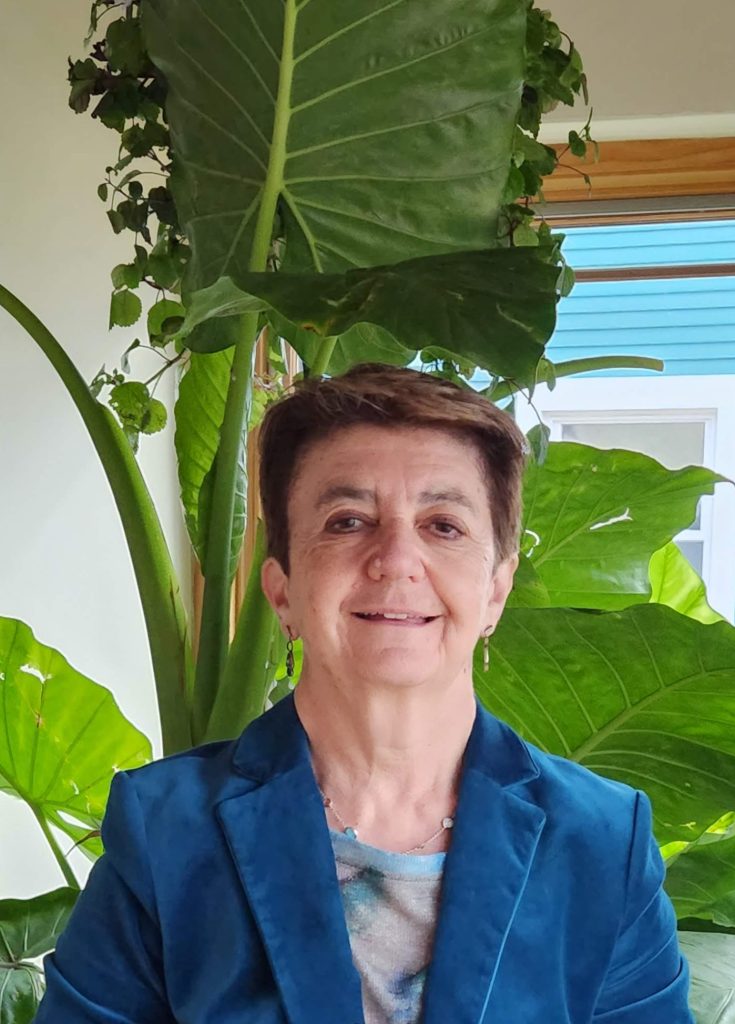
Emmanuel Dupoux
Textless NLP: towards language processing from raw audio.
Abstract: The oral (or gestural) modality is the most natural channel for human language interactions. Yet, language technology (Natural Language Processing, NLP) is primarily based on the written modality, and requires massive amounts of textual resources for the training of useful language models. As a result, even fundamentally speech-first applications like speech-to-speech translation or spoken assistants like Alexa, or Siri, are constructed in a Frankenstein way, with text as an intermediate representation between the signal and language models. Besides this being inefficient, This has two unfortunate consequences: first, only a small fraction of the world’s languages that have massive textual repositories can be addressed by current technology. Second, even for text-rich languages, the oral form mismatches the written form at a variety of levels, including vocabulary and expressions. The oral medium also contains typically unwritten linguistic features like rhythm and intonation (prosody) and rich paralinguistic information (non verbal vocalizations like laughter, cries, clicks, etc, nuances carried through changes in voice qualities) which are therefore inaccessible to language models. But is this a necessity? Could we build language applications directly from the audio stream without using any text? In this talk, we review recent breakthroughs in representation learning and self-supervised techniques which have made it possible to learn latent linguistic units directly from audio which unlock the learning of generative language models without the use of any text. We show that these models can capture heretofore unaddressed nuances of the oral language including in a dialogue context, opening up the possibility of speech-to-speech textless NLP applications. We outline existing technical challenges to achieve this goal, including challenges to build expressive oral language datasets at scale.
Short Bio: E. Dupoux is professor at the Ecole des Hautes Etudes en Sciences Sociales (EHESS) and Research Scientist at Meta AI Labs. He directs the Cognitive Machine Learning team at the Ecole Normale Supérieure (ENS) in Paris and INRIA. His education includes a PhD in Cognitive Science (EHESS), a MA in Computer Science (Orsay University) and a BA in Applied Mathematics (Pierre & Marie Curie University). His research mixes developmental science, cognitive neuroscience, and machine learning, with a focus on the reverse engineering of infant language and cognitive development using unsupervised or weakly supervised learning. He is the recipient of an Advanced ERC grant, co-organizer of the Zero Ressource Speech Challenge series (2015–2021), the Intuitive Physics Benchmark (2019) and led in 2017 a Jelinek Summer Workshop at CMU on multimodal speech learning. He is a CIFAR LMB and a ELLIS Fellow. He has authored 150 articles in peer reviewed outlets in cognitive science and language technology.
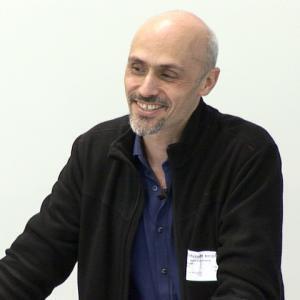
Elena Simperl
Knowledge graph use cases in natural language generation
Natural language generation (NLG) makes knowledge graphs (KGs) more accessible. I will present two applications of NLG in this space: in the first one, verbalisations of KG triples feed into downstream KG applications, allowing users with diverse levels of digital literacy to share their knowledge, and contribute to the KG. In the second one, having text representations of KG triples helps us verify the content of a KG against external sources towards more trustworthy KGs. I will present human-in-the-loop solutions to these applications that leverage a range of machine learning techniques to scale to the large, multilingual knowledge graphs modern applications use.
Short Bio: Elena Simperl is a Professor of Computer Science and Deputy Head of Department for Enterprise and Engagement in the Department of Informatics at King’s College London. She is also the Director of Research for the Open Data Institute (ODI) and a Fellow of the British Computer Society and the Royal Society of Arts. Elena features in the top 100 most influential scholars in knowledge engineering of the last decade. She obtained her doctoral degree in Computer Science from the Free University of Berlin, and her diploma in Computer Science from the Technical University of Munich. Prior to joining King’s in 2020, she was a Turing Fellow, and held positions in Germany, Austria and at the University of Southampton. Her research is at intersection between AI and social computing, helping designers understand how to build smart sociotechnical systems that combine data and algorithms with human and social capabilities. Elena led 14 European and national research projects, including recently QROWD, ODINE, Data Pitch, Data Stories, and ACTION. She is currently the scientific and technical director of MediaFutures, a Horizon 2020 programme that is using arts-inspired methods to design participatory AI systems that tackle misinformation and disinformation online. Elena’s interest in leading initiatives within the scientific community has also taken form through chairing several conferences in her field, including the European and International Semantic Web Conference series, the European Data Forum, and the European Semantic Technologies conference. She is the president of the Semantic Web Science Association.
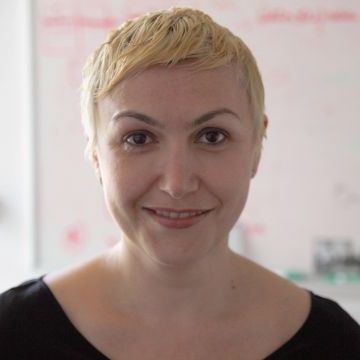
Ryan Lowe (remote)
Aligning ChatGPT: past, present, and future
In this talk I will present different perspectives on the alignment of chatbots like ChatGPT. I’ll review reinforcement learning from human feedback (RLHF), the core training technique behind InstructGPT and ChatGPT, including a brief history of how it was developed. I’ll discuss some of the pitfalls of RLHF, and what is being done today to address them. I’ll then speculate on some of the alignment challenges I expect we’ll face with this new generation of powerful personal assistants, how they could reshape society, and some things we’ll need to do to make sure these changes are good for humans.
Short Bio: Ryan is a researcher at OpenAI on the Alignment team. His most recent work involved proving out RLHF on language models, starting with summarization, then moving to InstructGPT and most recently ChatGPT and GPT-4. Previously, he worked on multi-agent RL, emergent communication, and dialogue systems at McGill University.
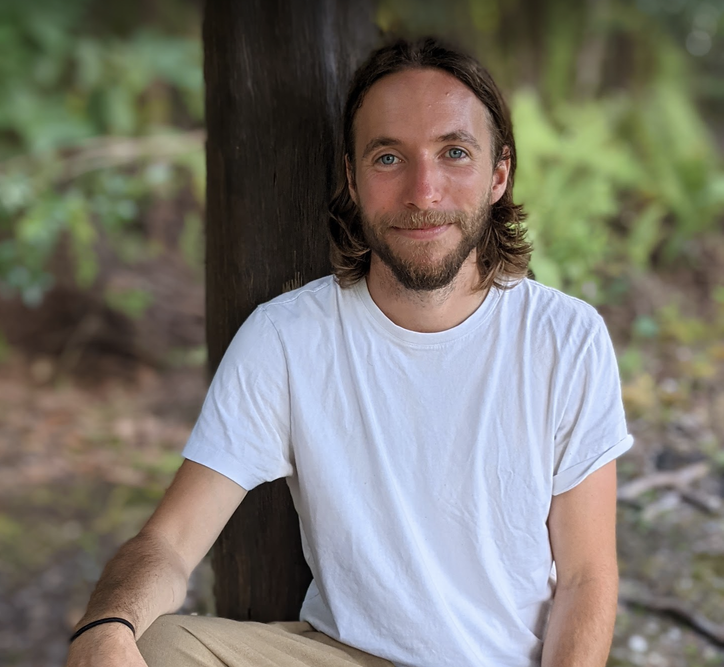